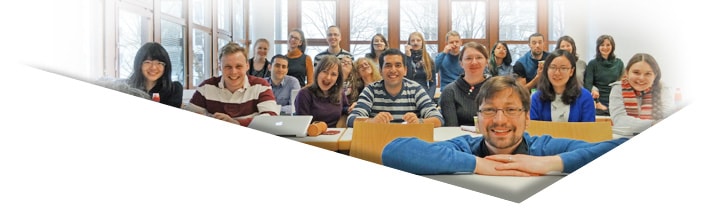
Bayesian Statistics and Inference
Standard parametrical and non- parametrical statistical tests implicitly assume large sample size and are based on frequentist approaches. However, especially in life science (and more so in medical trials and drug development), the sample size is small and the aim is actually deriving probability density distributions of parameters or odds ratios for competing models, rather than just giving p-values. If you work in the fields mentioned above, but don’t understand a single sentence of what is written here, this lecture is an absolute must-do for you!
The lecture is built up from Bayes‘ simple theorem and approaches statistical problems from the information theory point of view and thereby connects to graphs, diffusion maps, the basics of quantum mechanics and useful methods like the EM algorithm, maximum entropy, Occam’s Razor or the KL – divergence.
Topics:
- Bayes Theorem
- Information, Maximum Entropy and Lagrangian Multipliers
- Bayesian Parameter Estimation
- Model Selection
- Signal Detection
- Links to Graphs and Diffusion Maps
- Variational Bayes